Organizations are on the verge of losing control of their data forever
Who ever heard about the Data Team for the Data Team?
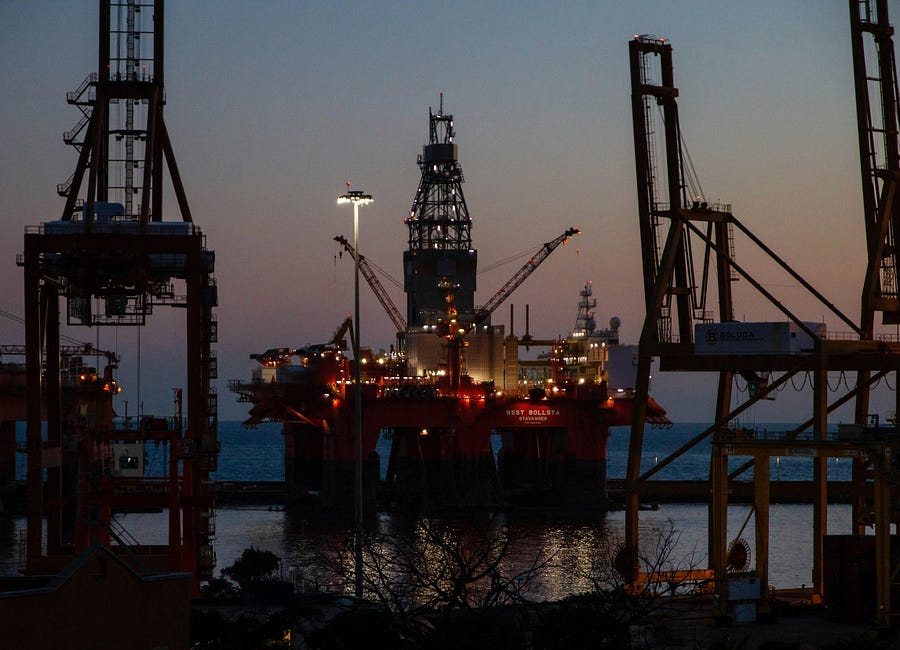
About me
I’m Hugo Lu — I started my career working in M&A in London before moving to JUUL and falling into data engineering. After a brief stint back in finance, I headed up the Data function at London-based Fintech Codat. I’m now CEO at Orchestra, which is a data release pipeline tool that helps Data Teams release data into production reliably and efficiently 🚀
Also check out our Substack and our internal blog ⭐️
Introduction
“Data is the new oil” — something we’re all completely sick and tired of hearing.
However, this phrase isn’t without justification. Good quality data, version-controlled data, and robust data has already revolutionised industries like quantitative finance.
With data-driven business processes, people working anywhere from marketing to support are also on their way to becoming more efficient. However many are still feeling left behind.
Consequently, we’ve seen huge numbers of layoffs in Data. Organisations frequently view data as a cost-centre rather than a value-driver.
Combined with the industry’s latest trend (AI), Organisations are on the verge of losing control of their Data Forever unless Data Teams can finally do some self-care, and in this article we’ll dive into why.
The Advent of Large Language Models (“LLMs”)
LLMs are really powerful. From simple chatbots to code-generation machines, LLMs are an exceptional addition to any productivity suite.
There are some clear applications of LLMs to Data Engineers and Analytics Engineers too — indeed, anyone seeking to maintain high data quality control standards. Controlling data was something we always used to struggle with in an operational context before LLMs.
For example, one thing I tried to do was to run a query every day that fetched every account, their follow-up dates, and compared this to the current date.
If it had been more than two weeks, I’d send an automated notification to the account owner, tagged in slack, tagged their manager, and ask them to update the record in Salesforce and follow-up.
This ended up being ignored, and it wasn’t helpful to account executives at all. This process was missing something important extremely important: context.
Sure — the follow-up date might be wrong but you could tell in the notes that they’d spoken more recently (so the alert was actually wrong). This meant people didn’t follow the alerts as they were missing context in unstructured data.
Now, with an LLM, you can reliably tell people in “interaction-based” work what their biggest takeaways might be for the day, or week. Simply update your vector store with all the latest correspondence, retrain the LLM, and ask it questions like “What do I need to do today?” and “What are the interesting things that happened in my sector I should be aware of?”
This is a far more effective and elegant solution to the problem I was trying to solve, and it will be enabled by generative AI.
Where’s the Data Quality Control?
Organisations frequently miss out on Data Quality Control. In the example above, the notes and the data was sufficiently good that my LLM knew what to do.
Often, this isn’t so. As organisations speed towards mass adoptions of LLMs, they’re unwittingly training them on terrible data.
You can see the excitement building. Companies like DataVolo, Instill.ai, or even vector stores like Unstructured are raising money at a clip, but the efforts to actually clean the stored data (all that is within pdfs, documents and so on) remain low.
Version controlling data, looking after big data, draining the data swamp, are rarely priorities. Organisations are increasingly preferring a focus on pushing data directly into cloud data warehouses and enterprise data catalogues, without first thinking about the use-cases and processes underpinning those datasets.
This creates a huge mess (c.f. Data Mess not Data Mesh), and puts organizations at risk of losing control of their data forever. If data is not cleaned before it is used to train LLMs, the cost of implementing generative AI will significantly increase as multiple retraining steps will be required as data quality issues thwart efforts to implement Gen AI.
What can we do?
The answer is to move more slowly — there is a large corpus of information available to enterprises. Some of this is helpful, and worth cleaning, some is not.
Before thinking about implementing LLMs, we should ask ourselves “What data is most valuable to our organisation?”.
Those datasets that are valuable, should be vetted. The processes, pipelines, and Orchestration should be made watertight. The data itself should be audited.
Only after doing this will Organizations realistically be in a position to not only make the most of their existing data, but they’ll also be ready to ensure data quality in the future. 💸
Find out more about Orchestra
Orchestra is a platform for getting the most value out of your data as humanely possible. It’s also a feature-rich orchestration tool, and can solve for multiple use-cases and solutions. Our docs are here, but why not also check out our integrations — we manage these so you can get started with your pipelines instantly. We also have a blog, written by the Orchestra team + guest writers, and some whitepapers for more in-depth reads.